
-
六扇门6123987.com点击有惊喜!
005期:必中七肖:多少买点.包您赚钱!
005期:必中五肖:重点多压
005期:必中㈢肖:稳准狠
005期:必中㈡肖:每期在这里和你相约
005期:必中㈠肖:【点击微信提前 免费领取】
005期:必中⑧码:【点击微信提前 免费领取】
-
六扇门6123987.com点击有惊喜!
004期:必中七肖:牛兔龙猴羊狗鼠
004期:必中五肖:牛兔龙猴羊
004期:必中㈢肖:牛兔龙
004期:必中㈡肖:牛兔
004期:必中㈠肖:牛
004期:必中⑧码:16.38.37.33.22.19.29.40
-
六扇门6123987.com点击有惊喜!
003期:必中七肖:蛇马猴鸡猪虎兔
003期:必中五肖:蛇马猴鸡猪
003期:必中㈢肖:蛇马猴
003期:必中㈡肖:蛇马
003期:必中㈠肖:蛇
003期:必中⑧码:12.3533.44.30.15.38.47
-
六扇门6123987.com点击有惊喜!
002期:必中七肖:虎龙蛇马猴鸡鼠
002期:必中五肖:虎龙蛇马猴
002期:必中㈢肖:虎龙蛇
002期:必中㈡肖:虎龙
002期:必中㈠肖:虎
002期:必中⑧码:15.37.24.35.09.44.29.39
-
六扇门6123987.com点击有惊喜!
001期:必中七肖:牛兔蛇羊猴狗鼠
001期:必中五肖:牛兔蛇羊猴
001期:必中㈢肖:牛兔蛇
001期:必中㈡肖:牛兔
001期:必中㈠肖:牛
001期:必中⑧码:28.38.1222.45.19.41.05
-
六扇门6123987.com点击有惊喜!
366期:必中七肖:鸡猪牛龙蛇羊鼠
366期:必中五肖:鸡猪牛龙蛇
366期:必中㈢肖:鸡猪牛
366期:必中㈡肖:鸡猪
366期:必中㈠肖:鸡
366期:必中⑧码:20.06.04.37.48.22.41.44
-
六扇门6123987.com点击有惊喜!
365期:必中七肖:兔狗猪牛虎马猴
365期:必中五肖:兔狗猪牛虎
365期:必中㈢肖:兔狗猪
365期:必中㈡肖:兔狗
365期:必中㈠肖:兔
365期:必中⑧码:26.19.30.28.39.47.09.02
-
六扇门6123987.com点击有惊喜!
364期:必中七肖:龙蛇马鸡猪牛兔
364期:必中五肖:龙蛇马鸡猪
364期:必中㈢肖:龙蛇马
364期:必中㈡肖:龙蛇
364期:必中㈠肖:龙
364期:必中⑧码:37.01.48.23.20.06.40.14
-
六扇门6123987.com点击有惊喜!
363期:必中七肖:鼠牛兔蛇猴羊猪
363期:必中五肖:鼠牛兔蛇猴
363期:必中㈢肖:鼠牛兔
363期:必中㈡肖:鼠牛
363期:必中㈠肖:鼠
363期:必中⑧码:17.04.26.48.09.22.18.41
-
六扇门6123987.com点击有惊喜!
362期:必中七肖:猴蛇鼠兔马龙羊
362期:必中五肖:猴蛇鼠兔马
362期:必中㈢肖:猴蛇鼠
362期:必中㈡肖:猴蛇
362期:必中㈠肖:猴
362期:必中⑧码:21.45.36.17.14.47.25.46
-
六扇门6123987.com点击有惊喜!
361期:必中七肖:狗蛇马羊鸡牛虎
361期:必中五肖:狗蛇马羊鸡
361期:必中㈢肖:狗蛇马
361期:必中㈡肖:狗蛇
361期:必中㈠肖:狗
361期:必中⑧码:07.36.47.10.20.40.03.31
-
六扇门6123987.com点击有惊喜!
360期:必中七肖:兔狗鼠蛇羊龙猪
360期:必中五肖:兔狗鼠蛇羊
360期:必中㈢肖:兔狗鼠
360期:必中㈡肖:兔狗
360期:必中㈠肖:兔
360期:必中⑧码:38.14.43.17.12.46.13.06
-
六扇门6123987.com点击有惊喜!
359期:必中七肖:鼠虎龙马猴鸡狗
359期:必中五肖:鼠虎龙马猴
359期:必中㈢肖:鼠虎龙
359期:必中㈡肖:鼠虎
359期:必中㈠肖:鼠
359期:必中⑧码:05.29.15.37.23.45.20.19
-
六扇门6123987.com点击有惊喜!
358期:必中七肖:牛兔蛇马狗猴鼠
358期:必中五肖:牛兔蛇马狗
358期:必中㈢肖:牛兔蛇
358期:必中㈡肖:牛兔
358期:必中㈠肖:牛
358期:必中⑧码:04.02.26.36.11.32.21.41
-
六扇门6123987.com点击有惊喜!
357期:必中七肖:猴牛兔蛇马鸡狗
357期:必中五肖:猴牛兔蛇马
357期:必中㈢肖:猴牛兔
357期:必中㈡肖:猴牛
357期:必中㈠肖:猴
357期:必中⑧码:21.40.14.36.47.08.31.16
-
六扇门6123987.com点击有惊喜!
356期:必中七肖:猪鼠蛇羊猴鸡狗
356期:必中五肖:猪鼠蛇羊猴
356期:必中㈢肖:猪鼠蛇
356期:必中㈡肖:猪鼠
356期:必中㈠肖:猪
356期:必中⑧码:06.29.26.46.44.20.07.42
-
六扇门6123987.com点击有惊喜!
355期:必中七肖:牛龙马猴鸡猪兔
355期:必中五肖:牛龙马猴鸡
355期:必中㈢肖:牛龙马
355期:必中㈡肖:牛龙
355期:必中㈠肖:牛
355期:必中⑧码:04.49.35.21.44.07.15.28
-
六扇门6123987.com点击有惊喜!
354期:必中七肖:猴猪龙蛇马羊牛
354期:必中五肖:猴猪龙蛇马羊
354期:必中㈢肖:猴猪龙
354期:必中㈡肖:猴猪
354期:必中㈠肖:猴
354期:必中⑧码:09.18.25.36.47.22.16.45
-
六扇门6123987.com点击有惊喜!
353期:必中七肖:狗马羊兔蛇鼠猴
353期:必中五肖:狗马羊兔蛇
353期:必中㈢肖:狗马羊
353期:必中㈡肖:狗马
353期:必中㈠肖:狗
353期:必中⑧码:19.47.22.38.24.05.45.43
-
六扇门6123987.com点击有惊喜!
352期:必中七肖:鼠虎羊蛇马狗猪
352期:必中五肖:鼠虎羊蛇马
352期:必中㈢肖:鼠虎羊
352期:必中㈡肖:鼠虎
352期:必中㈠肖:鼠
352期:必中⑧码:05.27.12.35.07.30.46.34
-
六扇门6123987.com点击有惊喜!
351期:必中七肖:牛龙马猴狗鼠虎
351期:必中五肖:牛龙马猴狗
351期:必中㈢肖:牛龙马
351期:必中㈡肖:牛龙
351期:必中㈠肖:牛
351期:必中⑧码:04.25.23.33.45.07.41.39
期数 | 三肖 | 特码 | 开奖号 | 结果 |
---|---|---|---|---|
005期 | 获取资料 | 获取资料 | 获取资料 | 获取资料 |
004期 | 兔-龙-猴 | 26-37-33 | 猴33 | 精准 |
003期 | 蛇-马-猴 | 12-35-33 | 猴33 | 精准 |
002期 | 鼠-虎-龙 | 05-27-37 | 龙37 | 精准 |
001期 | 蛇羊-狗 | 12-22-31 | 羊22 | 精准 |
366期 | 龙-猪-狗 | 37-06-43 | 猪06 | 精准 |
365期 | 蛇-猴-猪 | 12-33-30 | 猪30 | 精准 |
364期 | 虎-龙-猴 | 15-01-21 | 龙01 | 精准 |
363期 | 牛-蛇-狗 | 04-24-43 | 牛04 | 精准 |
362期 | 兔-猪-蛇 | 02-30-24 | 兔02 | 精准 |
361期 | 兔-羊鸡 | 26-46-20 | 鸡20 | 精准 |
360期 | 狗-兔-蛇 | 43-14-36 | 兔14 | 精准 |
359期 | 虎-羊-鸡 | 15-22-44 | 虎15 | 精准 |
358期 | 兔-猴-狗 | 38-21-24 | 猴21 | 精准 |
357期 | 猴-猪-蛇 | 09-42-24 | 猴09 | 精准 |
356期 | 虎-蛇-羊 | 27-24-46 | 羊46 | 精准 |
355期 | 龙-马-猴 | 49-11-45 | 龙49 | 精准 |
354期 | 羊-龙-鸡 | 46-25-20 | 龙25 | 精准 |
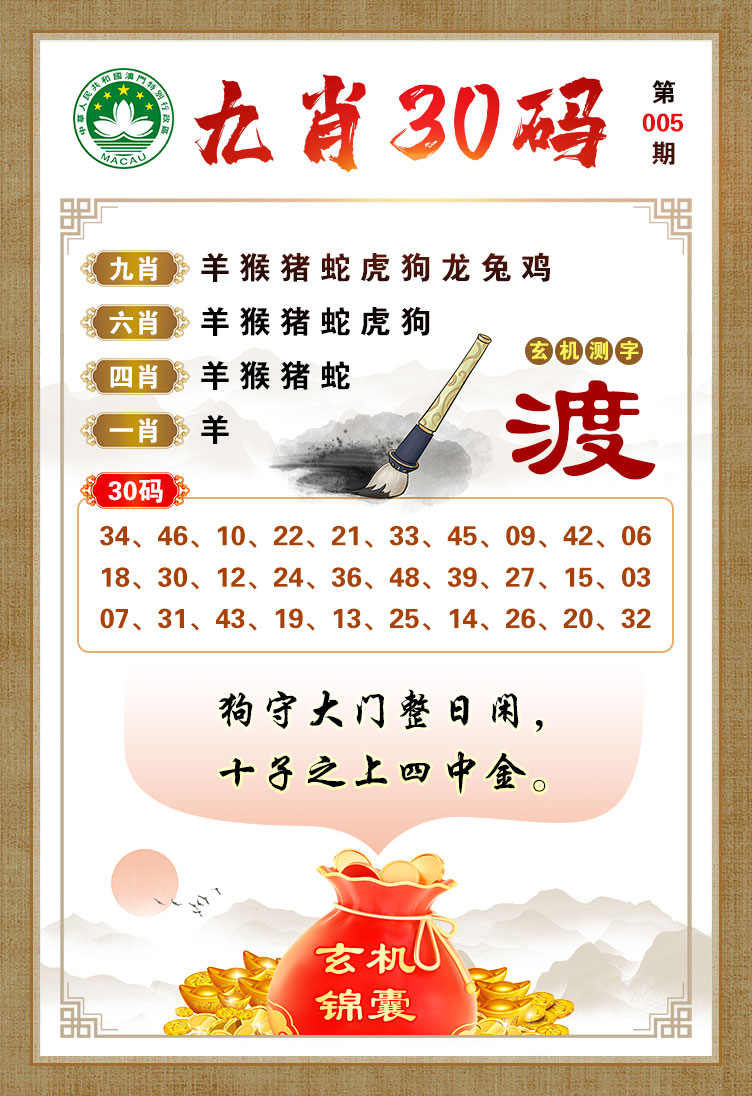
-
005期家野中特【更新】特开:?00准
-
004期家野中特【野兽】特开:猴33准
-
003期家野中特【野兽】特开:猴33准
-
002期家野中特【野兽】特开:龙37准
-
001期家野中特【家禽】特开:羊22准
-
366期家野中特【家禽】特开:猪06准
-
365期家野中特【家禽】特开:猪30准
-
364期家野中特【野兽】特开:龙01准
-
363期家野中特【家禽】特开:牛04准
-
362期家野中特【野兽】特开:兔02准
-
361期家野中特【家禽】特开:鸡20准
-
360期家野中特【野兽】特开:兔14准
-
359期家野中特【野兽】特开:虎15准
-
358期家野中特【野兽】特开:猴21准
-
357期家野中特【野兽】特开:猴09准
-
356期家野中特【家禽】特开:羊46准
-
355期家野中特【野兽】特开:龙49准
-
354期家野中特【野兽】特开:龙25准
-
353期家野中特【家禽】特开:马47准
-
352期家野中特【家禽】特开:羊10准
-
351期家野中特【野兽】特开:虎27准
-
350期家野中特【家禽】特开:牛04准
-
349期家野中特【野兽】特开:龙01准
-
348期家野中特【野兽】特开:鼠17准
-
347期家野中特【家禽】特开:牛28准
-
346期家野中特【野兽】特开:鼠41准
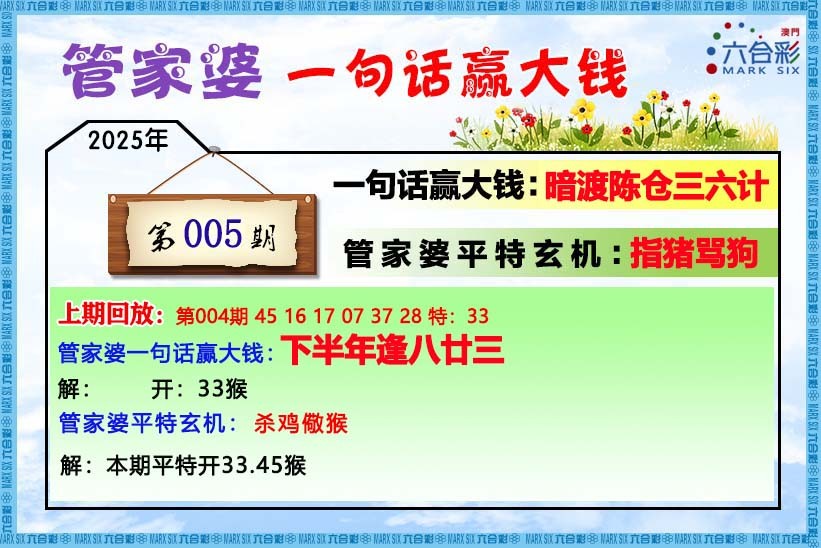
-
005期:双波中特【正在】【更新】开?00中
-
004期:双波中特【红波】【绿波】开猴33中
-
003期:双波中特【蓝波】【绿波】开猴33中
-
002期:双波中特【红波】【蓝波】开龙37中
-
001期:双波中特【红波】【绿波】开羊22中
-
366期:双波中特【蓝波】【绿波】开猪06中
-
365期:双波中特【红波】【蓝波】开猪30中
-
364期:双波中特【红波】【绿波】开龙01中
-
363期:双波中特【红波】【蓝波】开牛04中
-
362期:双波中特【红波】【绿波】开兔02中
-
361期:双波中特【红波】【蓝波】开鸡20中
-
360期:双波中特【蓝波】【绿波】开兔14中
-
359期:双波中特【蓝波】【红波】开虎15中
-
358期:双波中特【红波】【绿波】开猴21中
-
357期:双波中特【蓝波】【绿波】开猴09中
-
356期:双波中特【红波】【蓝波】开羊46中
-
355期:双波中特【红波】【绿波】开龙49中
-
354期:双波中特【蓝波】【红波】开龙25中
-
353期:双波中特【绿波】【蓝波】开马47中
-
352期:双波中特【蓝波】【红波】开羊10中
-
351期:双波中特【红波】【绿波】开虎27中
-
350期:双波中特【红波】【蓝波】开牛04中
-
349期:双波中特【蓝波】【红波】开龙01中
-
004期:六肖中特(资料开奖日更新)开?00中
-
004期:六肖中特(牛兔龙猴羊狗)开猴33中
-
003期:六肖中特(兔龙马猴鸡牛)开猴33中
-
002期:六肖中特(鼠虎龙马鸡猪)开龙37中
-
001期:六肖中特(龙蛇羊猴狗猪)开羊22中
-
366期:六肖中特(鼠牛龙蛇猴猪)开猪06中
-
365期:六肖中特(兔蛇羊狗猪牛)开猪30中
-
364期:六肖中特(牛虎龙马猴狗)开龙01中
-
363期:六肖中特(牛虎龙鸡马狗)开牛04中
-
362期:六肖中特(牛兔龙马猴狗)开兔02中
-
361期:六肖中特(牛兔龙猴鸡狗)开鸡20中
-
360期:六肖中特(鼠虎兔龙蛇猪)开兔14中
-
359期:六肖中特(虎龙羊鸡猪鼠)开虎15中
-
358期:六肖中特(牛兔马猴狗鼠)开猴21中
-
357期:六肖中特(猴狗虎马龙蛇)开猴09中
-
356期:六肖中特(猪鼠兔马羊鸡)开羊46中
-
355期:六肖中特(牛龙马猴鸡猪)开龙49中
-
354期:六肖中特(鼠虎龙马鸡猪)开龙25中
-
353期:六肖中特(牛兔龙马猴狗)开马47中
-
352期:六肖中特(羊猪牛虎龙马)开羊10中
-
351期:六肖中特(牛虎龙猴羊鸡)开虎27中
-
349期:六肖中特(龙兔虎狗马蛇)开龙01中
-
348期:六肖中特(马蛇龙兔虎鼠)开鼠17中
-
346期:六肖中特(羊猪马鼠蛇狗)开鼠41中
-
344期:六肖中特(兔虎鼠狗猴蛇)开兔02中
-
342期:六肖中特(龙兔虎鼠猴蛇)开龙49中
-
341期:六肖中特(猪狗鸡猴羊马)开猪18中
-
340期:六肖中特(牛猪狗鸡羊马)开马47中
-
339期:六肖中特(龙兔虎鼠羊马)开兔38中
-
337期:六肖中特(龙兔虎鼠猪蛇)开蛇48中
-
005期:琴棋书画(更新中)开?00中
-
004期:琴棋书画(棋书画)开猴33中
-
003期:琴棋书画(琴棋画)开猴33中
-
002期:琴棋书画(棋书画)开龙37中
-
001期:琴棋书画(琴书画)开羊22中
-
366期:琴棋书画(棋书画)开猪06中
-
365期:琴棋书画(琴书画)开猪30中
-
364期:琴棋书画(琴棋书)开龙01中
-
363期:琴棋书画(棋书画)开牛04中
-
362期:琴棋书画(琴棋书)开兔02中
-
361期:琴棋书画(琴书画)开鸡20中
-
360期:琴棋书画(琴棋画)开兔14中
-
359期:琴棋书画(棋书画)开虎15中
-
358期:琴棋书画(琴棋画)开猴21中
-
357期:琴棋书画(琴书画)开猴09中
-
356期:琴棋书画(琴书画)开羊46中
-
355期:琴棋书画(棋琴书)开龙49中
-
354期:琴棋书画(棋书画)开龙25中
-
353期:琴棋书画(琴棋书)开马47中
-
352期:琴棋书画(琴书画)开羊10中
-
351期:琴棋书画(琴棋书)开虎27中
-
350期:琴棋书画(棋书画)开牛04中
-
琴:【兔蛇鸡】
棋:【鼠牛狗】
书:【虎龙马】
画:【羊猴猪】
-
天=兔马猴猪牛龙
地=蛇羊鸡狗鼠虎
-
005期【正在更新】特开:?00准
-
004期【天肖天肖】特开:猴33准
-
003期【天肖天肖】特开:猴33准
-
002期【天肖天肖】特开:龙37准
-
001期【地肖地肖】特开:羊22准
-
366期【天肖天肖】特开:猪06准
-
365期【天肖天肖】特开:猪30准
-
364期【天肖天肖】特开:龙01准
-
363期【天肖天肖】特开:牛04准
-
362期【天肖天肖】特开:兔02准
-
361期【地肖地肖】特开:鸡20准
-
360期【天肖天肖】特开:兔14准
-
359期【地肖地肖】特开:虎15准
-
358期【天肖天肖】特开:猴21准
-
357期【天肖天肖】特开:猴09准
-
356期【地肖地肖】特开:羊46准
-
355期【天肖天肖】特开:龙49准
-
354期【天肖天肖】特开:龙25准
-
353期【天肖天肖】特开:马47准
-
352期【地肖地肖】特开:羊10准
-
351期【地肖地肖】特开:虎27准
-
349期【天肖天肖】特开:龙01准
-
347期【天肖天肖】特开:牛28准
-
345期【地肖地肖】特开:羊46准
-
005期:春夏秋冬(更新中)开?00中
-
004期:春夏秋冬(夏秋冬)开猴33中
-
003期:春夏秋冬(春秋冬)开猴33中
-
002期:春夏秋冬(春夏秋)开龙37中
-
001期:春夏秋冬(夏秋冬)开羊22中
-
366期:春夏秋冬(春夏冬)开猪06中
-
365期:春夏秋冬(春秋冬)开猪30中
-
364期:春夏秋冬(春夏冬)开龙01中
-
363期:春夏秋冬(夏秋冬)开牛04中
-
362期:春夏秋冬(春夏冬)开兔02中
-
361期:春夏秋冬(春秋冬)开鸡20中
-
360期:春夏秋冬(春夏秋)开兔14中
-
359期:春夏秋冬(春秋冬)开虎15中
-
358期:春夏秋冬(夏秋冬)开猴21中
-
357期:春夏秋冬(春夏秋)开猴09中
-
356期:春夏秋冬(春夏秋)开羊46中
-
355期:春夏秋冬(春夏秋)开龙49中
-
354期:春夏秋冬(春夏冬)开龙25中
-
353期:春夏秋冬(夏秋冬)开马47中
-
352期:春夏秋冬(春夏冬)开羊10中
-
351期:春夏秋冬(春秋冬)开虎27中
-
349期:春夏秋冬(春夏秋)开龙01中
-
346期:春夏秋冬(春秋冬)开鼠41中
-
345期:春夏秋冬(夏秋冬)开羊46中
-
344期:春夏秋冬(春夏秋)开兔02中
-
343期:春夏秋冬(春秋冬)开猴45中
-
342期:春夏秋冬(春夏秋)开龙49中
-
341期:春夏秋冬(春秋冬)开猪18中
-
340期:春夏秋冬(夏秋冬)开马47中
-
【春】兔、虎、龙
【夏】马、蛇、羊
【秋】鸡、猴、狗
【冬】鼠、猪、牛
-
九肖6123987.com这个网站不错开:?00准
-
八肖六扇门论坛开:?00准
-
六肖想提前看资料请加微信开:?00准
-
五肖开:?00准
-
四肖开:?00准
-
三肖开:?00准
-
二肖加微信免费领取资料开:?00准
-
一肖跟本站,迟早开路虎开:?00准
-
①码每期在这里和你相约开:?00准
-
③码每期在这里和你相约开:?00准
-
⑤码
每期在这里和你相约
开:?00准 -
⑩码每期在这里和你相约开:?00准
-
九肖兔狗马虎龙蛇羊牛鼠开:龙01准
-
八肖兔狗马虎龙蛇羊牛开:龙01准
-
六肖兔狗马虎龙蛇开:龙01准
-
五肖兔狗马虎龙开:龙01准
-
四肖兔狗马虎开:龙01准
-
三肖兔狗马开:龙01准
-
二肖兔狗开:龙01准
-
一肖兔开:龙01准
-
①码26开:龙01准
-
③码26.31.11开:龙01准
-
⑤码
26.31.11.15.01
开:龙01准 -
⑩码26.31.11.15.01
36.10.16.29.14
开:龙01准
-
九肖蛇马猴龙兔狗虎鼠牛开:鼠17准
-
八肖蛇马猴龙兔狗虎鼠开:鼠17准
-
六肖蛇马猴龙兔狗开:鼠17准
-
五肖蛇马猴龙兔开:鼠17准
-
四肖蛇马猴龙开:鼠17准
-
三肖蛇马猴开:鼠17准
-
二肖蛇马开:鼠17准
-
一肖蛇开:鼠17准
-
①码24开:鼠17准
-
③码24.23.09开:鼠17准
-
⑤码
24.23.09.01.14
开:鼠17准 -
⑩码24.23.09.01.14
31.15.17.16.36
开:鼠17准
-
九肖兔牛狗猪蛇虎羊鸡鼠开:牛28准
-
八肖兔牛狗猪蛇虎羊鸡开:牛28准
-
六肖兔牛狗猪蛇虎开:牛28准
-
五肖兔牛狗猪蛇开:牛28准
-
四肖兔牛狗猪开:牛28准
-
三肖兔牛狗开:牛28准
-
二肖兔牛开:牛28准
-
一肖兔开:牛28准
-
①码14开:牛28准
-
③码14.04.07开:牛28准
-
⑤码
14.04.07.18.12
开:牛28准 -
⑩码14.04.07.18.12
15.10.08.29.26
开:牛28准
-
九肖鼠猪马蛇鸡猴羊狗牛开:鼠41准
-
八肖鼠猪马蛇鸡猴羊狗开:鼠41准
-
六肖鼠猪马蛇鸡猴开:鼠41准
-
五肖鼠猪马蛇鸡开:鼠41准
-
四肖鼠猪马蛇开:鼠41准
-
三肖鼠猪马开:鼠41准
-
二肖鼠猪开:鼠41准
-
一肖鼠开:鼠41准
-
①码17开:鼠41准
-
③码17.30.11开:鼠41准
-
⑤码
17.30.11.24.08
开:鼠41准 -
⑩码17.30.11.24.08
21.10.43.28.29
开:鼠41准
-
九肖兔马虎狗蛇鸡羊龙猴开:兔02准
-
八肖兔马虎狗蛇鸡羊龙开:兔02准
-
六肖兔马虎狗蛇鸡开:兔02准
-
五肖兔马虎狗蛇开:兔02准
-
四肖兔马虎狗开:兔02准
-
三肖兔马虎开:兔02准
-
二肖兔马开:兔02准
-
一肖兔开:兔02准
-
①码26开:兔02准
-
③码26.35.39开:兔02准
-
⑤码
26.35.39.19.48
开:兔02准 -
⑩码26.35.39.19.48
08.34.13.33.38
开:兔02准
-
九肖龙猴虎兔狗蛇牛猪鼠开:龙49准
-
八肖龙猴虎兔狗蛇牛猪开:龙49准
-
六肖龙猴虎兔狗蛇开:龙49准
-
五肖龙猴虎兔狗开:龙49准
-
四肖龙猴虎兔开:龙49准
-
三肖龙猴虎开:龙49准
-
二肖龙猴开:龙49准
-
一肖龙开:龙49准
-
①码37开:龙49准
-
③码37.27.33开:龙49准
-
⑤码
37.27.33.26.31
开:龙49准 -
⑩码37.27.33.26.31
36.28.30.17.39
开:龙49准
-
九肖狗羊猪猴鸡蛇鼠马牛开:猪18准
-
八肖狗羊猪猴鸡蛇鼠马开:猪18准
-
六肖狗羊猪猴鸡蛇开:猪18准
-
五肖狗羊猪猴鸡开:猪18准
-
四肖狗羊猪猴开:猪18准
-
三肖狗羊猪开:猪18准
-
二肖狗羊开:猪18准
-
一肖狗开:猪18准
-
①码19开:猪18准
-
③码19.46.30开:猪18准
-
⑤码
19.46.30.33.08
开:猪18准 -
⑩码19.46.30.33.08
12.29.47.04.07
开:猪18准
-
九肖狗猪马牛鸡羊龙虎猴开:马47准
-
八肖狗猪马牛鸡羊龙虎开:马47准
-
六肖狗猪马牛鸡羊开:马47准
-
五肖狗猪马牛鸡开:马47准
-
四肖狗猪马牛开:马47准
-
三肖狗猪马开:马47准
-
二肖狗猪开:马47准
-
一肖狗开:马47准
-
①码07开:马47准
-
③码07.18.35开:马47准
-
⑤码
07.18.35.40.20
开:马47准 -
⑩码07.18.35.40.20
46.01.15.45.31
开:马47准
-
九肖兔鼠虎羊龙狗马猪牛开:兔38准
-
八肖兔鼠虎羊龙狗马猪开:兔38准
-
六肖兔鼠虎羊龙狗开:兔38准
-
五肖兔鼠虎羊龙开:兔38准
-
四肖兔鼠虎羊开:兔38准
-
三肖兔鼠虎开:兔38准
-
二肖兔鼠开:兔38准
-
一肖兔开:兔38准
-
①码02开:兔38准
-
③码02.05.39开:兔38准
-
⑤码
02.05.39.10.13
开:兔38准 -
⑩码02.05.39.10.13
31.23.42.28.26
开:兔38准
-
005期:绿蓝绿肖(正在更新)开?00中
-
004期:绿蓝绿肖(红肖蓝肖)开猴33中
-
003期:绿蓝绿肖(蓝肖绿肖)开猴33中
-
002期:绿蓝绿肖(红肖绿肖)开龙37中
-
001期:绿蓝绿肖(蓝肖绿肖)开羊22中
-
366期:绿蓝绿肖(红肖蓝肖)开猪06中
-
365期:绿蓝绿肖(蓝肖绿肖)开猪30中
-
364期:绿蓝绿肖(红肖绿肖)开龙01中
-
363期:绿蓝绿肖(蓝肖绿肖)开牛04中
-
362期:绿蓝绿肖(蓝肖红肖)开兔02中
-
361期:绿蓝绿肖(红肖绿肖)开鸡20中
-
360期:绿蓝绿肖(红肖蓝肖)开兔14中
-
359期:绿蓝绿肖(蓝肖绿肖)开虎15中
-
358期:绿蓝绿肖(红肖蓝肖)开猴21中
-
357期:绿蓝绿肖(蓝肖绿肖)开猴09中
-
356期:绿蓝绿肖(蓝肖绿肖)开羊46中
-
355期:绿蓝绿肖(红肖绿肖)开龙49中
-
354期:绿蓝绿肖(蓝肖绿肖)开龙25中
-
353期:绿蓝绿肖(红肖绿肖)开马47中
-
352期:绿蓝绿肖(绿肖红肖)开羊10中
-
351期:绿蓝绿肖(蓝肖绿肖)开虎27中
-
350期:绿蓝绿肖(蓝肖绿肖)开牛04中
-
349期:绿蓝绿肖(红肖绿肖)开龙01中
-
347期:绿蓝绿肖(红肖绿肖)开牛28中
-
346期:绿蓝绿肖(红肖蓝肖)开鼠41中
-
345期:绿蓝绿肖(红肖绿肖)开羊46中
-
红肖:马、兔、鼠、鸡
蓝肖:蛇、虎、猪、猴
绿肖:羊、龙、牛、狗
-
005期:〖更新中〗开?00准
-
004期:〖牛龙猴〗开猴33准
-
003期:〖兔蛇猴〗开猴33准
-
002期:〖鼠兔龙〗开龙37准
-
001期:〖蛇羊猴〗开羊22准
-
366期:〖龙蛇猪〗开猪06准
-
365期:〖兔羊猪〗开猪30准
-
364期:〖虎龙猴〗开龙01准
-
363期:〖兔马牛〗开牛04准
-
362期:〖牛兔猴〗开兔02准
-
361期:〖虎羊鸡〗开鸡20准
-
360期:〖羊鼠兔〗开兔14准
-
359期:〖虎龙羊〗开虎15准
-
358期:〖兔猴狗〗开猴21准
-
357期:〖虎蛇猴〗开猴09准
-
356期:〖羊鸡牛〗开羊46准
-
355期:〖牛龙猴〗开龙49准
-
354期:〖龙羊鸡〗开龙25准
-
353期:〖龙马猪〗开马47准
-
352期:〖羊牛狗〗开羊10准
-
351期:〖马兔虎〗开虎27准
-
349期:〖兔龙马〗开龙01准
-
346期:〖鼠马猪〗开鼠41准
-
344期:〖兔马虎〗开兔02准
-
342期:〖猴鼠龙〗开龙49准
-
341期:〖猪狗羊〗开猪18准
-
339期:〖鼠虎兔〗开兔38准
-
005期:菜肉草肖(正在更新)开?00中
-
004期:菜肉草肖(肉肖菜肖)开猴33中
-
003期:菜肉草肖(草肖菜肖)开猴33中
-
002期:菜肉草肖(肉肖菜肖)开龙37中
-
001期:菜肉草肖(菜肖草肖)开羊22中
-
366期:菜肉草肖(肉肖菜肖)开猪06中
-
365期:菜肉草肖(菜肖草肖)开猪30中
-
364期:菜肉草肖(肉肖草肖)开龙01中
-
363期:菜肉草肖(菜肖草肖)开牛04中
-
362期:菜肉草肖(肉肖草肖)开兔02中
-
361期:菜肉草肖(肉肖菜肖)开鸡20中
-
360期:菜肉草肖(菜肖草肖)开兔14中
-
359期:菜肉草肖(肉肖草肖)开虎15中
-
358期:菜肉草肖(菜肖肉肖)开猴21中
-
357期:菜肉草肖(菜肖草肖)开猴09中
-
356期:菜肉草肖(肉肖草肖)开羊46中
-
355期:菜肉草肖(菜肖肉肖)开龙49中
-
354期:菜肉草肖(肉肖草肖)开龙25中
-
353期:菜肉草肖(菜肖草肖)开马47中
-
352期:菜肉草肖(肉肖草肖)开羊10中
-
351期:菜肉草肖(菜肖肉肖)开虎27中
-
350期:菜肉草肖(肉肖草肖)开牛04中
-
349期:菜肉草肖(肉肖草肖)开龙01中
-
347期:菜肉草肖(菜肖草肖)开牛28中
-
346期:菜肉草肖(菜肖草肖)开鼠41中
-
345期:菜肉草肖(肉肖草肖)开羊46中
-
344期:菜肉草肖(草肖肉肖)开兔02中
-
342期:菜肉草肖(肉肖菜肖)开龙49中
-
341期:菜肉草肖(菜肖草肖)开猪18中
-
肉肖:虎蛇龙狗
菜肖:猪鼠鸡猴
草肖:牛羊马兔
-
005期:男女中特【正在】【更新】开猫00中
-
004期:男女中特【男肖】【男肖】开猴33中
-
003期:男女中特【男肖】【男肖】开猴33中
-
002期:男女中特【男肖】【男肖】开龙37中
-
001期:男女中特【女肖】【女肖】开羊22中
-
366期:男女中特【女肖】【女肖】开猪06中
-
365期:男女中特【女肖】【女肖】开猪30中
-
364期:男女中特【男肖】【男肖】开龙01中
-
363期:男女中特【男肖】【男肖】开牛04中
-
362期:男女中特【女肖】【女肖】开兔02中
-
361期:男女中特【女肖】【女肖】开鸡20中
-
360期:男女中特【女肖】【女肖】开兔14中
-
359期:男女中特【男肖】【男肖】开虎15中
-
358期:男女中特【男肖】【男肖】开猴21中
-
357期:男女中特【男肖】【男肖】开猴09中
-
356期:男女中特【女肖】【女肖】开羊46中
-
355期:男女中特【男肖】【男肖】开龙49中
-
354期:男女中特【男肖】【男肖】开龙25中
-
353期:男女中特【男肖】【男肖】开马47中
-
352期:男女中特【女肖】【女肖】开羊10中
-
351期:男女中特【男肖】【男肖】开虎27中
-
女肖:兔蛇羊鸡猪
男肖:鼠牛虎龙马猴狗
-
005期:六扇门平特(更新中)开00中
-
004期:六扇门平特(狗狗狗)开07中
-
003期:六扇门平特(马马马)开47中
-
002期:六扇门平特(牛牛牛)开04中
-
001期:六扇门平特(猪猪猪)开18中
-
366期:六扇门平特(蛇蛇蛇)开24中
-
365期:六扇门平特(鼠鼠鼠)开05中
-
364期:六扇门平特(虎虎虎)开15中
-
363期:六扇门平特(蛇蛇蛇)开36中
-
362期:六扇门平特(鼠鼠鼠)开05中
-
361期:六扇门平特(羊羊羊)开22中
-
360期:六扇门平特(马马马)开35中
-
359期:六扇门平特(兔兔兔)开02中
-
358期:六扇门平特(狗狗狗)开19中
-
357期:六扇门平特(牛牛牛)开16中
-
356期:六扇门平特(狗狗狗)开43中
-
355期:六扇门平特(猴猴猴)开09中
-
354期:六扇门平特(猪猪猪)开06中
-
353期:六扇门平特(牛牛牛)开40中
-
352期:六扇门平特(猴猴猴)开09中
-
351期:六扇门平特(羊羊羊)开22中
-
349期:六扇门平特(龙龙龙)开01中
-
005期:一行中特(金)防(木水土)开?00中
-
004期:一行中特(金)防(水火土)开猴33中
-
003期:一行中特(金)防(木水火)开猴33中
-
002期:一行中特(金)防(木火土)开龙37中
-
001期:一行中特(金)防(木水火)开羊22中
-
366期:一行中特(金)防(木水土)开猪06中
-
365期:一行中特(金)防(木水火)开猪30中
-
364期:一行中特(金)防(水火土)开龙01中
-
363期:一行中特(金)防(木水土)开牛04中
-
362期:一行中特(金)防(火水土)开兔02中
-
361期:一行中特(金)防(木水土)开鸡20中
-
360期:一行中特(金)防(木火土)开兔14中
-
359期:一行中特(金)防(木水火)开虎15中
-
358期:一行中特(金)防(木水土)开猴21中
-
357期:一行中特(金)防(木水火)开猴09中
-
356期:一行中特(金)防(木火土)开羊46中
-
355期:一行中特(金)防(木水土)开龙49中
-
354期:一行中特(金)防(木火土)开龙25中
-
353期:一行中特(金)防(水火土)开马47中
-
352期:一行中特(金)防(木火土)开羊10中
-
351期:一行中特(金)防(木火土)开虎27中
-
349期:一行中特(金)防(木水火)开龙01中
-
348期:一行中特(金)防(木火土)开鼠17中
-
347期:一行中特(金)防(木水火)开牛28中
-
346期:一行中特(金)防(水火土)开鼠41中
-
345期:一行中特(金)防(木水火)开羊46中
-
344期:一行中特(金)防(水火木)开兔02中
-
343期:一行中特(金)防(木水火)开猴45中
-
340期:一行中特(金)防(水土火)开马47中
-
339期:一行中特(金)防(水火土)开兔38中
-
338期:一行中特(金)防(水木土)开牛40中
-
【金】:02 03 10 11 24 25 32 33 40 41
【木】:06 07 14 15 22 23 36 37 44 45
【水】:12 13 20 21 28 29 42 43
【火】:01 08 09 16 17 30 31 38 39 46 47
【土】:04 05 18 19 26 27 34 35 48 49
-
005期杀特三肖[更新中]开?00中
-
004期杀特三肖[蛇马鸡]开猴33中
-
003期杀特三肖[鼠羊狗]开猴33中
-
002期杀特三肖[牛羊狗]开龙37中
-
001期杀特三肖[鼠虎马]开羊22中
-
366期杀特三肖[虎兔马]开猪06中
-
365期杀特三肖[虎龙鸡]开猪30中
-
364期杀特三肖[鼠兔羊]开龙01中
-
363期杀特三肖[龙蛇鸡]开牛04中
-
362期杀特三肖[虎马鸡]开兔02中
-
361期杀特三肖[鼠龙马]开鸡20中
-
360期杀特三肖[牛虎鸡]开兔14中
-
359期杀特三肖[兔蛇鸡]开虎15中
-
358期杀特三肖[虎龙鸡]开猴21中
-
357期杀特三肖[鼠龙羊]开猴09中
-
356期杀特三肖[虎龙马]开羊46中
-
355期杀特三肖[蛇羊狗]开龙49中
-
354期杀特三肖[虎羊猪]开龙25中
-
353期杀特三肖[牛兔羊]开马47中
-
352期杀特三肖[鼠兔狗]开羊10中
-
351期杀特三肖[兔狗马]开虎27中
-
349期杀特三肖[鼠鸡猴]开龙01中
-
348期杀特三肖[羊牛鸡]开鼠17中
-
347期杀特三肖[鼠猴马]开牛28中
-
346期杀特三肖[兔龙虎]开鼠41中
-
345期杀特三肖[猴虎牛]开羊46中
-
344期杀特三肖[鼠牛猪]开兔02中
-
342期杀特三肖[猪鸡羊]开龙49中
-
341期杀特三肖[虎龙牛]开猪18中
-
005期杀特三尾[更新中]开?00中
-
004期杀特三尾[027尾]开猴33中
-
003期杀特三尾[167尾]开猴33中
-
002期杀特三尾[046尾]开龙37中
-
001期杀特三尾[158尾]开羊22中
-
366期杀特三尾[124尾]开猪06中
-
365期杀特三尾[367尾]开猪30中
-
364期杀特三尾[068尾]开龙01中
-
363期杀特三尾[156尾]开牛04中
-
362期杀特三尾[358尾]开兔02中
-
361期杀特三尾[146尾]开鸡20中
-
360期杀特三尾[238尾]开兔14中
-
359期杀特三尾[148尾]开虎15中
-
358期杀特三尾[247尾]开猴21中
-
357期杀特三尾[358尾]开猴09中
-
356期杀特三尾[247尾]开羊46中
-
355期杀特三尾[135尾]开龙49中
-
354期杀特三尾[237尾]开龙25中
-
353期杀特三尾[136尾]开马47中
-
352期杀特三尾[352尾]开羊10中
-
351期杀特三尾[128尾]开虎27中
-
350期杀特三尾[157尾]开牛04中
-
349期杀特三尾[074尾]开龙01中
-
348期杀特三尾[018尾]开鼠17中
-
347期杀特三尾[012尾]开牛28中
-
346期杀特三尾[048尾]开鼠41中
-
345期杀特半波[蓝单]开羊46中
-
346期杀特半波[绿双]开鼠41中
-
347期杀特半波[红双]开牛28中
-
348期杀特半波[红双]开鼠17中
-
349期杀特半波[绿单]开龙01中
-
350期杀特半波[绿单]开牛04中
-
351期杀特半波[蓝双]开虎27中
-
352期杀特半波[绿单]开羊10中
-
353期杀特半波[红双]开马47中
-
354期杀特半波[绿双]开龙25中
-
355期杀特半波[红双]开龙49中
-
356期杀特半波[蓝单]开羊46中
-
357期杀特半波[红双]开猴09中
-
358期杀特半波[蓝双]开猴21中
-
359期杀特半波[绿双]开虎15中
-
360期杀特半波[红单]开兔14中
-
361期杀特半波[绿单]开鸡20中
-
362期杀特半波[蓝单]开兔02中
-
363期杀特半波[红单]开牛04中
-
364期杀特半波[绿双]开龙01中
-
365期杀特半波[蓝单]开猪30中
-
366期杀特半波[红双]开猪06中
-
001期杀特半波[蓝单]开羊22中
-
002期杀特半波[红双]开龙37中
-
003期杀特半波[蓝双]开猴33中
-
004期杀特半波[红双]开猴33中
-
005期杀特半波[更新]开?00中
- 精品四不像
- 澳门挂牌
- 澳门跑狗图
- 九肖十码
- 女人味
- 美人鱼
- 澳门春宫圖
- 澳门跑马图
- 正版蛇蛋图
- 凤凰天机图
- 澳门傳眞
- 龙门客栈
- 男人味
- 澳门红虎
- 澳門内幕
- 相入非非
- 石獅镇碼
- 每日闲情
- 幽默猜测
- 功夫早茶
- 一句真言
- 周公解梦
- 趣味幽默
- 老版跑狗
- 特码诗句
- 另版跑狗
- 番薯真人
- 金钱豹A
- 澳彩签牌
- 值日生肖
- 通天报社
- 奥彩专用
- 奥客家娘
- 财神报A
- 银财神报
- 财神报B
- 金财神报
- 请财神报
- 蓝财神报
- 平财神报
- 财神报C
- 绿财神报
- 波贴天机
- 独解传真
- 澳门马票
- 金钱豹B
- 早茶论码
- 拾贰地支
- 妈祖显灵
- 六合神童
- 皇道吉日
- 澳门西游
- 玄机秘贴
- 正版射牌
- 平特一肖
- 凤凰卜卦
- 钟馗大神
- 正版诗象
- 心水彩报
- 青龙精解
- 宝马彩报
- 澳门日报
- 澳门信封
- 白狼经典
- 小幽默之
- 澳彩图库
- 秘典玄机
- 六合内幕
- 六合典家
- 财神特码
- 财童送宝
- 财童送码
- 内幕玄機
- 博彩特利
- 六合雄霸
- 龍騰豹變
- 内幕选馬
- 馬會追踪
- 馬會追踪
- 妈祖献宝
- 奇准无比
- 猪哥报
- 六合英雄
- 六合黑庄
- 急智金囊
- 香港挂牌
- 大富翁
- 神鸟版
- 发财献策
- 马经霸王
- 诸葛神算
- 发财波局
- 识破玄机
- 狗头报
- 蜗牛报
- 独一无二
- 会员料
- 玄学代码
- 五星报B
- 神算策略
- 大话特码
- 铁算盘
- 广东赌王
- 观音心经
- 六合飞鸽
- 藏宝图
- 精品报
- 天尊报
- 洋通报
- 特码图
- 天机报
- 内幕报
- 马会图
- 九龙密报
- 小贴士A
- 湖南码报
- 莲花报
- 九龙精解
- 三元神算
- 经典尾报
- 经典单双
- 不服来战
- 新大富翁
- 发财秘诀
- 红灯笼
- 码头诗
- 六合头条
- 蓝财神
- 电邮天地
- 女财神报
- 东南漫画
- 金元宝
- 赚客报
- 新粤彩4
- 天眼神算
- 香港挂牌
- 正手写A
- 白姐泄密
- 精锐报
- 一样发
- 五福临门
- 佛祖救世
- 内幕赌经
- 福星报
- 心水特码
- 孔明报
- 蛇报A
- 旺角菜报
- 三合皇
- 一封信
- 点将单
- 水果报
-
2024龙年(十二生肖号码对照)鼠
[冲马]
05172941牛[冲羊]
04162840虎[冲猴]
03152739兔[冲鸡]
02142638龙[冲狗]
0113253749蛇[冲猪]
12243648马[冲鼠]
11233547羊[冲牛]
10223446猴[冲虎]
09213345鸡[冲兔]
08203244狗[冲龙]
07193143猪[冲蛇]
06183042 -
玻色参照表红波:0102070812131819232429303435404546蓝波:03040910141520252631363741424748绿波:05061116172122272832333839434449
-
五行参照表金:02031011242532334041木:06071415222336374445水:1213202128294243火:0108091617303138394647土:04051819262734354849
-
合数单双合数单:01030507091012141618212325272930323436384143454749合数双:020406081113151719202224262831333537394042444648
-
生肖属性照表家禽:牛、马、羊、鸡、狗、猪野兽:鼠、虎、兔、龙、蛇、猴吉美:兔、龙、蛇、马、羊、鸡凶丑:鼠、牛、虎、猴、狗、猪阴性:鼠、龙、蛇、马、狗、猪阳性:牛、虎、兔、羊、猴、鸡单笔:鼠、龙、马、蛇、鸡、猪双笔:虎、猴、狗、兔、羊、牛天肖:兔、马、猴、猪、牛、龙地肖:蛇、羊、鸡、狗、鼠、虎白边:鼠、牛、虎、鸡、狗、猪黑中:兔、龙、蛇、马、羊、猴女肖:兔、蛇、羊、鸡、猪(五宫肖)男肖:鼠、牛、虎、龙、马、猴、狗三合:鼠龙猴、牛蛇鸡、虎马狗、兔羊猪六合:鼠牛、龙鸡、虎猪、蛇猴、兔狗、马羊琴:兔蛇鸡棋:鼠牛狗书:虎龙马画:羊猴猪五福肖::鼠、虎、兔、蛇、猴[龙]红肖:马、兔、鼠、鸡蓝肖:蛇、虎、猪、猴绿肖:羊、龙、牛、狗

-
六扇门6123987.com点击有惊喜!
002期:必中七肖:多少买点.包您赚钱!
002期:必中五肖:重点多压
002期:必中㈢肖:稳准狠
002期:必中㈡肖:每期在这里和你相约
002期:必中㈠肖:【点击微信提前免费领取】
002期:必中⑧码:【点击微信提前免费领取】
-
六扇门6123987.com点击有惊喜!
001期:必中七肖:虎蛇马羊鸡狗鼠
001期:必中五肖:虎蛇马羊鸡
001期:必中㈢肖:虎蛇马
001期:必中㈡肖:虎蛇
001期:必中㈠肖:虎
001期:必中⑧码:40.24.11.22.32.19.41.28
-
六扇门6123987.com点击有惊喜!
140期:必中七肖:鼠虎兔蛇羊狗牛
140期:必中五肖:鼠虎兔蛇羊
140期:必中㈢肖:鼠虎兔
140期:必中㈡肖:鼠虎
140期:必中㈠肖:鼠
140期:必中⑧码:41.15.14.36.10.43.28.17
-
六扇门6123987.com点击有惊喜!
139期:必中七肖:狗鼠兔马猴羊鸡
139期:必中五肖:狗鼠兔马猴
139期:必中㈢肖:狗鼠兔
139期:必中㈡肖:狗鼠
139期:必中㈠肖:狗
139期:必中⑧码:43.41.17.38.23.33.22.32
-
六扇门6123987.com点击有惊喜!
138期:必中七肖:猴狗猪兔龙马羊
138期:必中五肖:猴狗猪兔龙
138期:必中㈢肖:猴狗猪
138期:必中㈡肖:猴狗
138期:必中㈠肖:猴
138期:必中⑧码:45.19.18.26.49.35.10.07
-
六扇门6123987.com点击有惊喜!
137期:必中七肖:羊猪虎蛇猴鸡鼠
137期:必中五肖:羊猪虎蛇猴
137期:必中㈢肖:羊猪虎
137期:必中㈡肖:羊猪
137期:必中㈠肖:羊
137期:必中⑧码:22.18.39.12.10.45.08.41
-
六扇门6123987.com点击有惊喜!
136期:必中七肖:鼠兔牛猴狗鸡马
136期:必中五肖:鼠兔牛猴狗
136期:必中㈢肖:鼠兔牛
136期:必中㈡肖:鼠兔
136期:必中㈠肖:鼠
136期:必中⑧码:29.38.22.45.31.08.23.28
-
六扇门6123987.com点击有惊喜!
135期:必中七肖:猪龙鼠兔鸡猴猪
135期:必中五肖:猪龙鼠兔鸡
135期:必中㈢肖:猪龙鼠
135期:必中㈡肖:猪龙
135期:必中㈠肖:猪
135期:必中⑧码:18.30.13.29.26.44.25.42
-
六扇门6123987.com点击有惊喜!
134期:必中七肖:牛蛇羊狗猪兔马
134期:必中五肖:牛蛇羊狗猪
134期:必中㈢肖:牛蛇羊
134期:必中㈡肖:牛蛇
134期:必中㈠肖:牛
134期:必中⑧码:16.28.24.10.07.06.23.19
-
六扇门6123987.com点击有惊喜!
133期:必中七肖:猴鼠虎牛兔蛇龙
133期:必中五肖:猴鼠虎牛兔
133期:必中㈢肖:猴鼠虎
133期:必中㈡肖:猴鼠
133期:必中㈠肖:猴
133期:必中⑧码:21.17.39.04.38.36.40.37
-
六扇门6123987.com点击有惊喜!
132期:必中七肖:羊鸡猪狗猴蛇马
132期:必中五肖:羊鸡猪狗猴
132期:必中㈢肖:羊鸡猪
132期:必中㈡肖:羊鸡
132期:必中㈠肖:羊
132期:必中⑧码:22.20.18.19.45.24.35.30
-
六扇门6123987.com点击有惊喜!
131期:必中七肖:猪牛狗马鸡羊龙
131期:必中五肖:猪牛狗马鸡
131期:必中㈢肖:猪牛狗
131期:必中㈡肖:猪牛
131期:必中㈠肖:猪
131期:必中⑧码:30.04.19.23.08.34.13.18
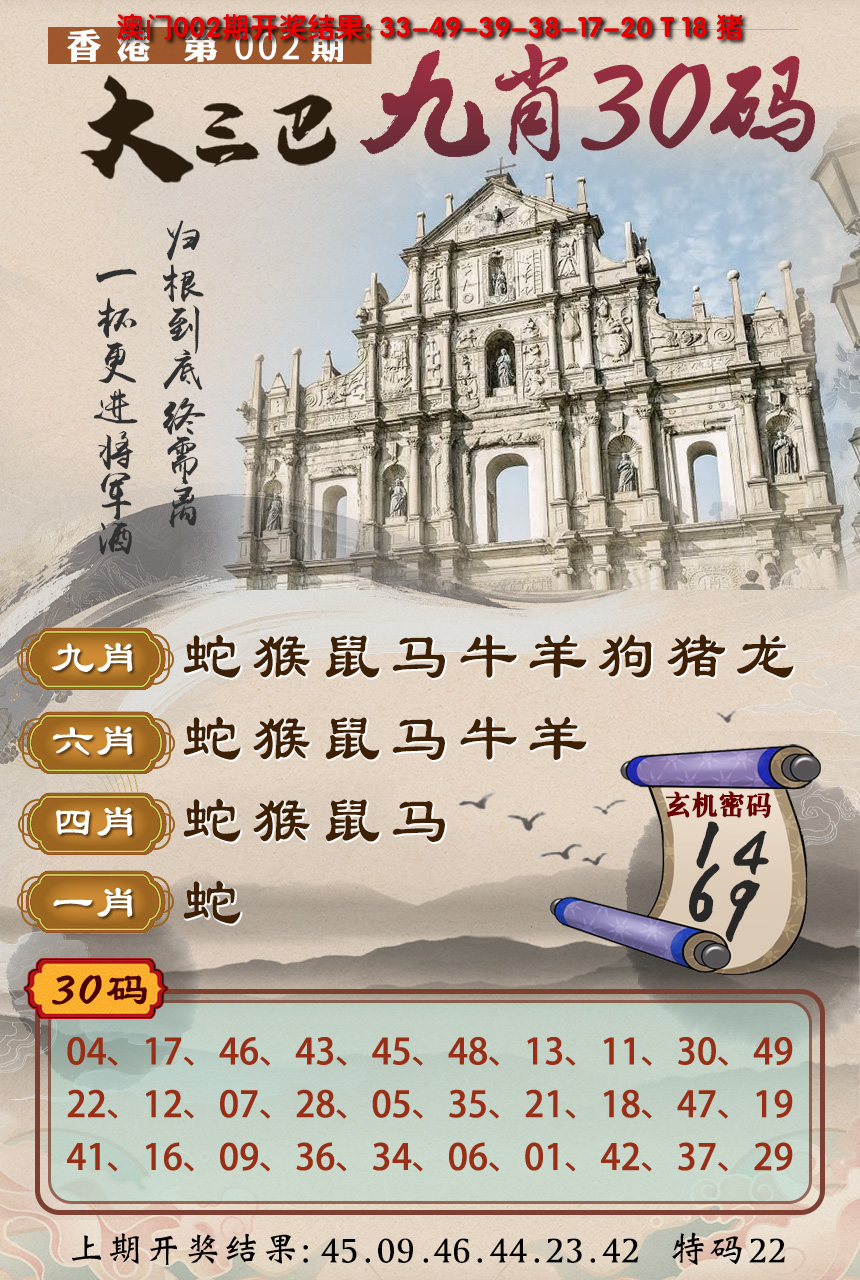
-
002期家野中特【更新】特开:?00准
-
001期家野中特【野兽】特开:羊22准
-
140期家野中特【野兽】特开:兔14准
-
139期家野中特【野兽】特开:猴45准
-
138期家野中特【家禽】特开:猪18准
-
137期家野中特【家禽】特开:羊10准
-
136期家野中特【家禽】特开:牛28准
-
135期家野中特【野兽】特开:兔38准
-
134期家野中特【家禽】特开:羊22准
-
133期家野中特【家禽】特开:牛04准
-
132期家野中特【家禽】特开:羊22准
-
131期家野中特【家禽】特开:羊22准
-
130期家野中特【家禽】特开:马23准
-
129期家野中特【野兽】特开:兔38准
-
128期家野中特【野兽】特开:龙01准
-
127期家野中特【家禽】特开:羊34准
-
126期家野中特【家禽】特开:马35准
-
125期家野中特【野兽】特开:蛇12准
-
124期家野中特【野兽】特开:鼠29准
-
123期家野中特【家禽】特开:马23准
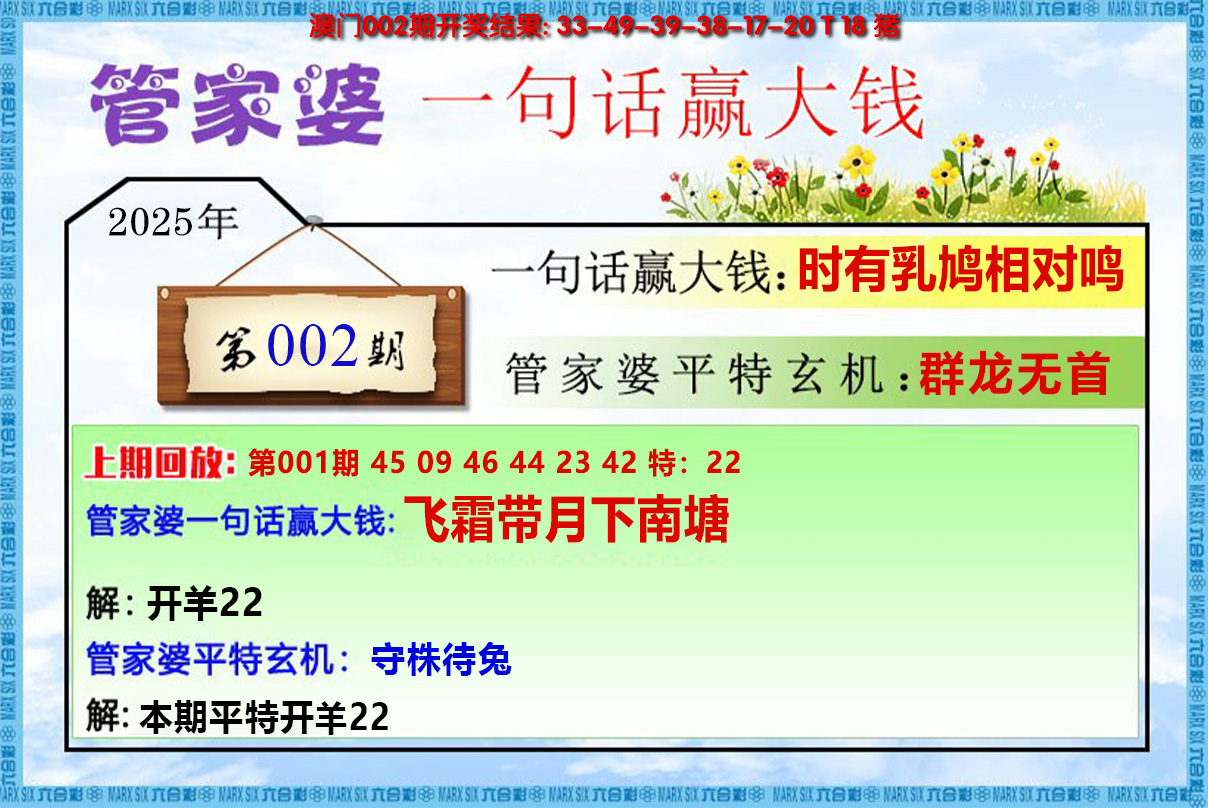
-
002期:双波中特【正在】【更新】开?00中
-
001期:双波中特【红波】【绿波】开羊22中
-
140期:双波中特【绿波】【蓝波】开兔14中
-
139期:双波中特【红波】【蓝波】开猴45中
-
138期:双波中特【红波】【绿波】开猪18中
-
137期:双波中特【蓝波】【绿波】开羊10中
-
136期:双波中特【绿波】【红波】开牛28中
-
135期:双波中特【绿波】【蓝波】开兔38中
-
134期:双波中特【绿波】【红波】开羊22中
-
002期:六肖中特(资料开奖日更新)开?00中
-
001期:六肖中特(虎蛇羊猴狗猪)开羊22中
-
140期:六肖中特(虎兔龙羊鸡猪)开兔14中
-
139期:六肖中特(鼠兔龙猴狗猪)开猴45中
-
138期:六肖中特(猪牛兔马羊狗)开猪18中
-
137期:六肖中特(蛇羊鸡猪虎兔)开羊10中
-
136期:六肖中特(狗猪牛兔龙马)开牛28中
-
135期:六肖中特(兔猪虎狗马蛇)开兔38中
-
134期:六肖中特(马蛇龙兔虎羊)开羊22中
-
133期:六肖中特(羊猪马牛蛇狗)开牛04中
-
132期:六肖中特(羊虎鼠狗猴蛇)开羊22中
-
131期:六肖中特(羊兔虎鼠猴蛇)开羊22中
-
130期:六肖中特(马狗鸡猴羊猪)开马23中
-
129期:六肖中特(牛猪狗鸡羊兔)开兔38中
-
128期:六肖中特(狗龙虎鼠羊马)开龙01中
-
127期:六肖中特(龙兔虎鼠猪羊)开羊34中
-
126期:六肖中特(龙兔鸡猴马蛇)开马35中
-
125期:六肖中特(虎牛狗蛇马羊)开蛇12中
-
002期:琴棋书画(更新中)开?00中
-
001期:琴棋书画(棋书画)开羊22中
-
140期:琴棋书画(琴棋书)开兔14中
-
139期:琴棋书画(琴书画)开猴45中
-
138期:琴棋书画(琴棋画)开猪18中
-
137期:琴棋书画(琴书画)开羊10中
-
136期:琴棋书画(琴棋画)开牛28中
-
135期:琴棋书画(琴书画)开兔38中
-
134期:琴棋书画(琴棋画)开羊22中
-
133期:琴棋书画(琴棋书)开牛04中
-
132期:琴棋书画(琴画书)开羊22中
-
131期:琴棋书画(画书琴)开羊22中
-
130期:琴棋书画(琴棋书)开马23中
-
129期:琴棋书画(画棋书)开兔38中
-
128期:琴棋书画(棋画书)开龙01中
-
127期:琴棋书画(琴画书)开羊34中
-
126期:琴棋书画(棋琴书)开马35中
-
125期:琴棋书画(琴书画)开蛇12中
-
124期:琴棋书画(琴棋画)开鼠29中
-
123期:琴棋书画(琴画书)开马23中
-
122期:琴棋书画(棋书画)开猪30中
-
琴:【兔蛇鸡】
棋:【鼠牛狗】
书:【虎龙马】
画:【羊猴猪】
-
天=兔马猴猪牛龙
地=蛇羊鸡狗鼠虎
-
002期【正在更新】特开:?00准
-
001期【地肖地肖】特开:羊22准
-
140期【天肖天肖】特开:兔14准
-
139期【天肖天肖】特开:猴45准
-
138期【天肖天肖】特开:猪18准
-
137期【地肖地肖】特开:羊10准
-
136期【天肖天肖】特开:牛28准
-
135期【天肖天肖】特开:兔38准
-
134期【地肖地肖】特开:羊22准
-
133期【天肖天肖】特开:牛04准
-
132期【地肖地肖】特开:羊22准
-
131期【地肖地肖】特开:羊22准
-
130期【天肖天肖】特开:马23准
-
002期:春夏秋冬(更新中)开?00中
-
001期:春夏秋冬(夏秋冬)开羊22中
-
140期:春夏秋冬(春夏冬)开兔14中
-
139期:春夏秋冬(夏秋冬)开猴45中
-
138期:春夏秋冬(春秋冬)开猪18中
-
137期:春夏秋冬(秋夏冬)开羊10中
-
136期:春夏秋冬(春秋冬)开牛28中
-
135期:春夏秋冬(春夏秋)开兔38中
-
134期:春夏秋冬(春秋夏)开羊22中
-
133期:春夏秋冬(冬秋夏)开牛04中
-
132期:春夏秋冬(夏春秋)开羊22中
-
131期:春夏秋冬(春夏冬)开羊22中
-
130期:春夏秋冬(夏春秋)开马23中
-
129期:春夏秋冬(秋冬春)开兔38中
-
128期:春夏秋冬(春秋冬)开龙01中
-
127期:春夏秋冬(夏春秋)开羊34中
-
126期:春夏秋冬(春冬夏)开马35中
-
125期:春夏秋冬(春夏秋)开蛇12中
-
124期:春夏秋冬(春冬秋)开鼠29中
-
123期:春夏秋冬(春秋夏)开马23中
-
【春】兔、虎、龙
【夏】马、蛇、羊
【秋】鸡、猴、狗
【冬】鼠、猪、牛
-
九肖6123987.com这个网站不错开:?00准
-
八肖六扇门论坛开:?00准
-
六肖想提前看资料请加微信开:?00准
-
五肖开:?00准
-
四肖开:?00准
-
三肖开:?00准
-
二肖加微信免费领取资料开:?00准
-
一肖跟本站,迟早开路虎开:?00准
-
①码每期在这里和你相约开:?00准
-
③码每期在这里和你相约开:?00准
-
⑤码
每期在这里和你相约
开:?00准 -
⑩码每期在这里和你相约开:?00准
-
九肖龙狗马虎兔蛇羊牛鼠开:兔38准
-
八肖龙狗马虎兔蛇羊牛开:兔38准
-
六肖龙狗马虎兔蛇开:兔38准
-
五肖龙狗马虎兔开:兔38准
-
四肖龙狗马虎开:兔38准
-
三肖龙狗马开:兔38准
-
二肖龙狗开:兔38准
-
一肖龙开:兔38准
-
①码26开:兔38准
-
③码26.31.11开:兔38准
-
⑤码
26.31.11.15.38
开:兔38准 -
⑩码26.31.11.15.38
36.10.16.29.14
开:兔38准
-
九肖蛇马猴龙兔狗虎羊牛开:羊22准
-
八肖蛇马猴龙兔狗虎羊开:羊22准
-
六肖蛇马猴龙兔狗开:羊22准
-
五肖蛇马猴龙兔开:羊22准
-
四肖蛇马猴龙开:羊22准
-
三肖蛇马猴开:羊22准
-
二肖蛇马开:羊22准
-
一肖蛇开:羊22准
-
①码24开:羊22准
-
③码24.23.09开:羊22准
-
⑤码
24.23.09.01.14
开:羊22准 -
⑩码24.23.09.01.14
31.15.22.16.36
开:羊22准
-
九肖兔牛狗猪蛇虎羊鸡鼠开:牛04准
-
八肖兔牛狗猪蛇虎羊鸡开:牛04准
-
六肖兔牛狗猪蛇虎开:牛04准
-
五肖兔牛狗猪蛇开:牛04准
-
四肖兔牛狗猪开:牛04准
-
三肖兔牛狗开:牛04准
-
二肖兔牛开:牛04准
-
一肖兔开:牛04准
-
①码14开:牛04准
-
③码14.28.07开:牛04准
-
⑤码
14.28.07.18.12
开:牛04准 -
⑩码14.28.07.18.12
15.10.08.29.26
开:牛04准
-
九肖羊猪马蛇鸡猴鼠狗牛开:羊22准
-
八肖羊猪马蛇鸡猴鼠狗开:羊22准
-
六肖羊猪马蛇鸡猴开:羊22准
-
五肖羊猪马蛇鸡开:羊22准
-
四肖羊猪马蛇开:羊22准
-
三肖羊猪马开:羊22准
-
二肖羊猪开:羊22准
-
一肖羊开:羊22准
-
①码17开:羊22准
-
③码17.30.11开:羊22准
-
⑤码
17.30.11.24.08
开:羊22准 -
⑩码17.30.11.24.08
21.10.43.28.29
开:羊22准
-
九肖羊马虎狗蛇鸡兔龙猴开:羊22准
-
八肖羊马虎狗蛇鸡兔龙开:羊22准
-
六肖羊马虎狗蛇鸡开:羊22准
-
五肖羊马虎狗蛇开:羊22准
-
四肖羊马虎狗开:羊22准
-
三肖羊马虎开:羊22准
-
二肖羊马开:羊22准
-
一肖羊开:羊22准
-
①码26开:羊22准
-
③码26.35.39开:羊22准
-
⑤码
26.35.39.19.48
开:羊22准 -
⑩码26.35.39.19.48
08.34.13.33.38
开:羊22准
-
九肖马猴虎兔狗蛇牛猪鼠开:马23准
-
八肖马猴虎兔狗蛇牛猪开:马23准
-
六肖马猴虎兔狗蛇开:马23准
-
五肖马猴虎兔狗开:马23准
-
四肖马猴虎兔开:马23准
-
三肖马猴虎开:马23准
-
二肖马猴开:马23准
-
一肖马开:马23准
-
①码37开:马23准
-
③码37.27.33开:马23准
-
⑤码
37.27.33.26.31
开:马23准 -
⑩码37.27.33.26.31
36.28.30.17.39
开:马23准
-
九肖狗羊兔猴鸡蛇鼠马牛开:兔38准
-
八肖狗羊兔猴鸡蛇鼠马开:兔38准
-
六肖狗羊兔猴鸡蛇开:兔38准
-
五肖狗羊兔猴鸡开:兔38准
-
四肖狗羊兔猴开:兔38准
-
三肖狗羊兔开:兔38准
-
二肖狗羊开:兔38准
-
一肖狗开:兔38准
-
①码19开:兔38准
-
③码19.46.30开:兔38准
-
⑤码
19.46.30.33.08
开:兔38准 -
⑩码19.46.30.33.08
12.29.47.04.07
开:兔38准
-
九肖狗猪龙牛鸡羊马虎猴开:龙01准
-
八肖狗猪龙牛鸡羊马虎开:龙01准
-
六肖狗猪龙牛鸡羊开:龙01准
-
五肖狗猪龙牛鸡开:龙01准
-
四肖狗猪龙牛开:龙01准
-
三肖狗猪龙开:龙01准
-
二肖狗猪开:龙01准
-
一肖狗开:龙01准
-
①码07开:龙01准
-
③码07.18.35开:龙01准
-
⑤码
07.18.35.40.20
开:龙01准 -
⑩码07.18.35.40.20
46.01.15.45.31
开:龙01准
-
九肖羊鼠虎兔龙狗马猪牛开:羊34准
-
八肖羊鼠虎兔龙狗马猪开:羊34准
-
六肖羊鼠虎兔龙狗开:羊34准
-
五肖羊鼠虎兔龙开:羊34准
-
四肖羊鼠虎兔开:羊34准
-
三肖羊鼠虎开:羊34准
-
二肖羊鼠开:羊34准
-
一肖羊开:羊34准
-
①码02开:羊34准
-
③码02.05.39开:羊34准
-
⑤码
02.05.39.10.13
开:羊34准 -
⑩码02.05.39.10.13
31.23.42.28.26
开:羊34准
-
002期:绿蓝绿肖(正在更新)开?00中
-
001期:绿蓝绿肖(蓝肖绿肖)开羊22中
-
140期:绿蓝绿肖(红肖绿肖)开兔14中
-
139期:绿蓝绿肖(蓝肖绿肖)开猴45中
-
138期:绿蓝绿肖(红肖蓝肖)开猪18中
-
137期:绿蓝绿肖(蓝肖绿肖)开羊10中
-
136期:绿蓝绿肖(红肖绿肖)开牛28中
-
135期:绿蓝绿肖(蓝肖红肖)开兔38中
-
134期:绿蓝绿肖(红肖绿肖)开羊22中
-
133期:绿蓝绿肖(绿肖红肖)开牛04中
-
132期:绿蓝绿肖(绿肖蓝肖)开羊22中
-
131期:绿蓝绿肖(红肖绿肖)开羊22中
-
130期:绿蓝绿肖(红肖绿肖)开马23中
-
129期:绿蓝绿肖(蓝肖红肖)开兔38中
-
128期:绿蓝绿肖(绿肖蓝肖)开龙01中
-
127期:绿蓝绿肖(红肖绿肖)开羊34中
-
126期:绿蓝绿肖(红肖绿肖)开马35中
-
125期:绿蓝绿肖(蓝肖绿肖)开蛇12中
-
124期:绿蓝绿肖(蓝肖红肖)开鼠29中
-
123期:绿蓝绿肖(蓝肖红肖)开马23中
-
122期:绿蓝绿肖(红肖蓝肖)开猪30中
-
121期:绿蓝绿肖(绿肖蓝肖)开狗07中
-
120期:绿蓝绿肖(绿肖红肖)开鼠17中
-
红肖:马、兔、鼠、鸡
蓝肖:蛇、虎、猪、猴
绿肖:羊、龙、牛、狗
-
002期:〖更新中〗开?00准
-
001期:〖虎蛇羊〗开羊22准
-
140期:〖兔龙羊〗开兔14准
-
139期:〖兔羊猴〗开猴45准
-
138期:〖牛马猪〗开猪18准
-
137期:〖虎羊鸡〗开羊10准
-
136期:〖牛兔狗〗开牛28准
-
135期:〖羊猪兔〗开兔38准
-
134期:〖羊马猪〗开羊22准
-
133期:〖牛马虎〗开牛04准
-
132期:〖猴鼠羊〗开羊22准
-
131期:〖羊狗猪〗开羊22准
-
130期:〖鼠虎马〗开马23准
-
002期:菜肉草肖(正在更新)开?00中
-
001期:菜肉草肖(肉肖草肖)开羊22中
-
140期:菜肉草肖(菜肖草肖)开兔14中
-
139期:菜肉草肖(肉肖菜肖)开猴45中
-
138期:菜肉草肖(菜肖草肖)开猪18中
-
137期:菜肉草肖(肉肖草肖)开羊10中
-
136期:菜肉草肖(草肖菜肖)开牛28中
-
135期:菜肉草肖(肉肖草肖)开兔38中
-
134期:菜肉草肖(草肖肉肖)开羊22中
-
133期:菜肉草肖(菜肖草肖)开牛04中
-
132期:菜肉草肖(草肖菜肖)开羊22中
-
131期:菜肉草肖(肉肖草肖)开羊22中
-
130期:菜肉草肖(草肖肉肖)开马23中
-
129期:菜肉草肖(草肖菜肖)开兔38中
-
128期:菜肉草肖(肉肖草肖)开龙01中
-
肉肖:虎蛇龙狗
菜肖:猪鼠鸡猴
草肖:牛羊马兔
-
002期:男女中特【正在】【更新】开猫00中
-
001期:男女中特【女肖】【女肖】开羊22中
-
140期:男女中特【女肖】【女肖】开兔14中
-
139期:男女中特【男肖】【男肖】开猴45中
-
138期:男女中特【女肖】【女肖】开猪18中
-
137期:男女中特【女肖】【女肖】开羊10中
-
136期:男女中特【男肖】【男肖】开牛28中
-
女肖:兔蛇羊鸡猪
男肖:鼠牛虎龙马猴狗
-
002期:六扇门平特(更新中)开00中
-
001期:六扇门平特(猴猴猴)开45中
-
140期:六扇门平特(龙龙龙)开01中
-
139期:六扇门平特(鼠鼠鼠)开41中
-
138期:六扇门平特(兔兔兔)开14中
-
137期:六扇门平特(虎虎虎)开03中
-
136期:六扇门平特(马马马)开11中
-
135期:六扇门平特(兔兔兔)开38中
-
002期:一行中特(金)防(木水土)开?00中
-
001期:一行中特(金)防(木火土)开羊22中
-
140期:一行中特(金)防(木水火)开兔14中
-
139期:一行中特(金)防(木火土)开猴45中
-
138期:一行中特(金)防(水火土)开猪18中
-
137期:一行中特(金)防(水火土)开羊10中
-
136期:一行中特(金)防(木水土)开牛28中
-
135期:一行中特(金)防(木水火)开兔38中
-
134期:一行中特(金)防(火木土)开羊22中
-
133期:一行中特(金)防(木土火)开牛04中
-
132期:一行中特(木)防(水火土)开羊22中
-
131期:一行中特(金)防(土水木)开羊22中
-
130期:一行中特(木)防(水火金)开马23中
-
129期:一行中特(金)防(火水木)开兔38中
-
128期:一行中特(金)防(水土火)开龙01中
-
127期:一行中特(金)防(水土火)开羊34中
-
126期:一行中特(土)防(水木金)开马35中
-
【金】:02 03 10 11 24 25 32 33 40 41
【木】:06 07 14 15 22 23 36 37 44 45
【水】:12 13 20 21 28 29 42 43
【火】:01 08 09 16 17 30 31 38 39 46 47
【土】:04 05 18 19 26 27 34 35 48 49
-
002期杀特三肖[更新中]开?00中
-
001期杀特三肖[牛兔龙]开羊22中
-
140期杀特三肖[鼠虎马]开兔14中
-
139期杀特三肖[牛蛇羊]开猴45中
-
138期杀特三肖[鼠龙羊]开猪18中
-
137期杀特三肖[兔蛇猴]开羊10中
-
136期杀特三肖[龙猴猪]开牛28中
-
135期杀特三肖[鼠鸡猴]开兔38中
-
134期杀特三肖[狗牛鸡]开羊22中
-
133期杀特三肖[鼠猴马]开牛04中
-
132期杀特三肖[兔龙虎]开羊22中
-
131期杀特三肖[猴虎牛]开羊22中
-
130期杀特三肖[鼠牛猪]开马23中
-
129期杀特三肖[猪鸡羊]开兔38中
-
128期杀特三肖[虎龙牛]开龙01中
-
127期杀特三肖[兔蛇鼠]开羊34中
-
126期杀特三肖[牛猴鸡]开马35中
-
125期杀特三肖[鸡马羊]开蛇12中
-
124期杀特三肖[鼠牛马]开鼠29中
-
123期杀特三肖[鸡猴龙]开马23中
-
122期杀特三肖[猴狗鸡]开猪30中
-
002期杀特三尾[更新中]开?00中
-
001期杀特三尾[158尾]开羊22中
-
140期杀特三尾[027尾]开兔14中
-
139期杀特三尾[237尾]开猴45中
-
138期杀特三尾[137尾]开猪18中
-
137期杀特三尾[357尾]开羊10中
-
136期杀特三尾[246尾]开牛28中
-
135期杀特三尾[157尾]开兔38中
-
134期杀特三尾[074尾]开羊22中
-
133期杀特三尾[018尾]开牛04中
-
132期杀特三尾[012尾]开羊22中
-
131期杀特三尾[048尾]开羊22中
-
130期杀特三尾[045尾]开马23中
-
129期杀特三尾[179尾]开兔38中
-
128期杀特三尾[024尾]开龙01中
-
127期杀特三尾[459尾]开羊34中
-
126期杀特三尾[349尾]开马35中
-
125期杀特三尾[035尾]开蛇12中
-
124期杀特三尾[467尾]开鼠29中
-
122期杀特半波[绿单]开猪30中
-
123期杀特半波[绿单]开马23中
-
124期杀特半波[蓝双]开鼠29中
-
125期杀特半波[绿单]开蛇12中
-
126期杀特半波[绿双]开马35中
-
127期杀特半波[红双]开羊34中
-
128期杀特半波[蓝双]开龙01中
-
129期杀特半波[蓝双]开兔38中
-
130期杀特半波[蓝单]开马23中
-
131期杀特半波[绿双]开羊22中
-
132期杀特半波[红双]开羊22中
-
133期杀特半波[红双]开牛04中
-
134期杀特半波[绿单]开羊22中
-
135期杀特半波[绿单]开兔38中
-
136期杀特半波[红双]开牛28中
-
137期杀特半波[绿单]开羊10中
-
138期杀特半波[蓝单]开猪18中
-
139期杀特半波[绿双]开猴45中
-
140期杀特半波[红单]开兔14中
-
001期杀特半波[蓝单]开羊22中
-
002期杀特半波[更新]开?00中
- 精品四不像
- 澳门挂牌
- 澳门跑狗图
- 九肖十码
- 女人味
- 美人鱼
- 澳门春宫圖
- 澳门跑马图
- 正版蛇蛋图
- 凤凰天机图
- 澳门傳眞
- 龙门客栈
- 男人味
- 澳门红虎
- 澳門内幕
- 相入非非
- 石獅镇碼
- 每日闲情
- 幽默猜测
- 功夫早茶
- 一句真言
- 周公解梦
- 趣味幽默
- 老版跑狗
- 特码诗句
- 另版跑狗
- 番薯真人
- 金钱豹A
- 澳彩签牌
- 值日生肖
- 通天报社
- 奥彩专用
- 奥客家娘
- 财神报A
- 银财神报
- 财神报B
- 金财神报
- 请财神报
- 蓝财神报
- 平财神报
- 财神报C
- 绿财神报
- 波贴天机
- 独解传真
- 澳门马票
- 金钱豹B
- 早茶论码
- 拾贰地支
- 妈祖显灵
- 六合神童
- 皇道吉日
- 澳门西游
- 玄机秘贴
- 正版射牌
- 平特一肖
- 凤凰卜卦
- 钟馗大神
- 正版诗象
- 心水彩报
- 青龙精解
- 宝马彩报
- 澳门日报
- 澳门信封
- 白狼经典
- 小幽默之
- 澳彩图库
- 秘典玄机
- 六合内幕
- 六合典家
- 财神特码
- 财童送宝
- 财童送码
- 内幕玄機
- 博彩特利
- 六合雄霸
- 龍騰豹變
- 内幕选馬
- 馬會追踪
- 馬會追踪
- 妈祖献宝
- 奇准无比
- 猪哥报
- 六合英雄
- 六合黑庄
- 急智金囊
- 香港挂牌
- 大富翁
- 神鸟版
- 发财献策
- 马经霸王
- 诸葛神算
- 发财波局
- 识破玄机
- 狗头报
- 蜗牛报
- 独一无二
- 会员料
- 玄学代码
- 五星报B
- 神算策略
- 大话特码
- 铁算盘
- 广东赌王
- 观音心经
- 六合飞鸽
- 藏宝图
- 精品报
- 天尊报
- 洋通报
- 特码图
- 天机报
- 内幕报
- 马会图
- 九龙密报
- 小贴士A
- 湖南码报
- 莲花报
- 九龙精解
- 三元神算
- 经典尾报
- 经典单双
- 不服来战
- 新大富翁
- 发财秘诀
- 红灯笼
- 码头诗
- 六合头条
- 蓝财神
- 电邮天地
- 女财神报
- 东南漫画
- 金元宝
- 赚客报
- 新粤彩4
- 天眼神算
- 香港挂牌
- 正手写A
- 白姐泄密
- 精锐报
- 一样发
- 五福临门
- 佛祖救世
- 内幕赌经
- 福星报
- 心水特码
- 孔明报
- 蛇报A
- 旺角菜报
- 三合皇
- 一封信
- 点将单
- 水果报
-
2024龙年(十二生肖号码对照)鼠
[冲马]
05172941牛[冲羊]
04162840虎[冲猴]
03152739兔[冲鸡]
02142638龙[冲狗]
0113253749蛇[冲猪]
12243648马[冲鼠]
11233547羊[冲牛]
10223446猴[冲虎]
09213345鸡[冲兔]
08203244狗[冲龙]
07193143猪[冲蛇]
06183042 -
玻色参照表红波:0102070812131819232429303435404546蓝波:03040910141520252631363741424748绿波:05061116172122272832333839434449
-
五行参照表金:02031011242532334041木:06071415222336374445水:1213202128294243火:0108091617303138394647土:04051819262734354849
-
合数单双合数单:01030507091012141618212325272930323436384143454749合数双:020406081113151719202224262831333537394042444648
-
生肖属性照表家禽:牛、马、羊、鸡、狗、猪野兽:鼠、虎、兔、龙、蛇、猴吉美:兔、龙、蛇、马、羊、鸡凶丑:鼠、牛、虎、猴、狗、猪阴性:鼠、龙、蛇、马、狗、猪阳性:牛、虎、兔、羊、猴、鸡单笔:鼠、龙、马、蛇、鸡、猪双笔:虎、猴、狗、兔、羊、牛天肖:兔、马、猴、猪、牛、龙地肖:蛇、羊、鸡、狗、鼠、虎白边:鼠、牛、虎、鸡、狗、猪黑中:兔、龙、蛇、马、羊、猴女肖:兔、蛇、羊、鸡、猪(五宫肖)男肖:鼠、牛、虎、龙、马、猴、狗三合:鼠龙猴、牛蛇鸡、虎马狗、兔羊猪六合:鼠牛、龙鸡、虎猪、蛇猴、兔狗、马羊琴:兔蛇鸡棋:鼠牛狗书:虎龙马画:羊猴猪五福肖::鼠、虎、兔、蛇、猴[龙]红肖:马、兔、鼠、鸡蓝肖:蛇、虎、猪、猴绿肖:羊、龙、牛、狗